Crop Mapping and Field Boundary Detection
Agricultural Research Center (ARC)
Business Needs
Agriculture automation is a main concern and an emerging subject for every country to optimize providing and reserving the annual storage of food and to solve problems facing the agriculture sector, such as crop disease management, pest control, weed management, irrigation, and water management. Agricultural Research Center (ARC) wanted to proactively support the increase of vegetation production in different regions all over Egypt. The vision was to identify the various vegetation types/crops using AI and remote sensing to automate the recommendation of the appropriate crops suitable for each land types (e.g., moisture levels of soil or droughty). The customer also wanted a more efficient automated process to identify planted areas, and hence having a better way to predict the required amount of irrigation water and the expected crop yield. This also entailed a need for raising the efficiency of ARC staff by building their capacity to use the latest technology for rapid crop type identification and field boundary detection. ARC also wanted to enable farmer’s engagement through reporting their field observations.
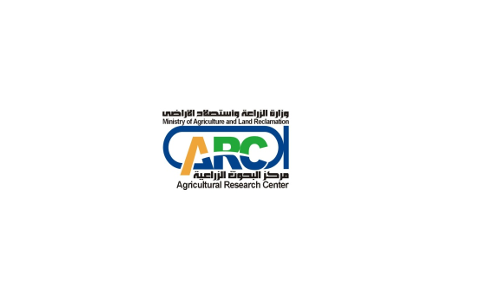
Golden Points
- Crop detection enables more accurate yield estimation, and hence better monitoring of crop production and distribution.
- Crop detection enables better estimation of irrigation water consumption, and hence better control of irrigation water loss.
- Better understanding of the cultivated areas enables a more efficient crop production planning.
Used Software
- ArcGIS Pro installed on a data science virtual machine (DSVM) image from Microsoft
- ArcGIS Enterprise
- Portal for ArcGIS
- ArcGIS Pro
- Spatial Analyst extension
- Surevy123
- Workforce for ArcGIS
- Collector for ArcGIS
- Operations Dashboard for ArcGIS